A Review of The Landscape and What’s on the Horizon¹
In the early detection of prostate cancer (PCa), prostate MRI continues to play a leading role. Assessing risk with MRI can make an important difference in the diagnosis and has an equally important impact on PI-RADS evaluations.
Updated in 2019, the Prostate Imaging Reporting and Data System (PI-RADS) was first introduced in 2012 to provide radiologists and urologists a “more standardized acquisition, interpretation, and reporting of prostate MRI.”
Thanks to the PI-RADS system, radiologists use the analyses and information provided by multiparametric-MRI sources to evaluate lesions by giving them a PI-RADS score, which can range from 1 to 5, based on the likelihood of the lesion to be clinically significant cancer. In lesions with PI-RADS scores higher than 3, a biopsy is generally indicated. Here are the 5 PI-RADS scores and what they mean:
PI‑RADS SCORES: BY THE NUMBERS1
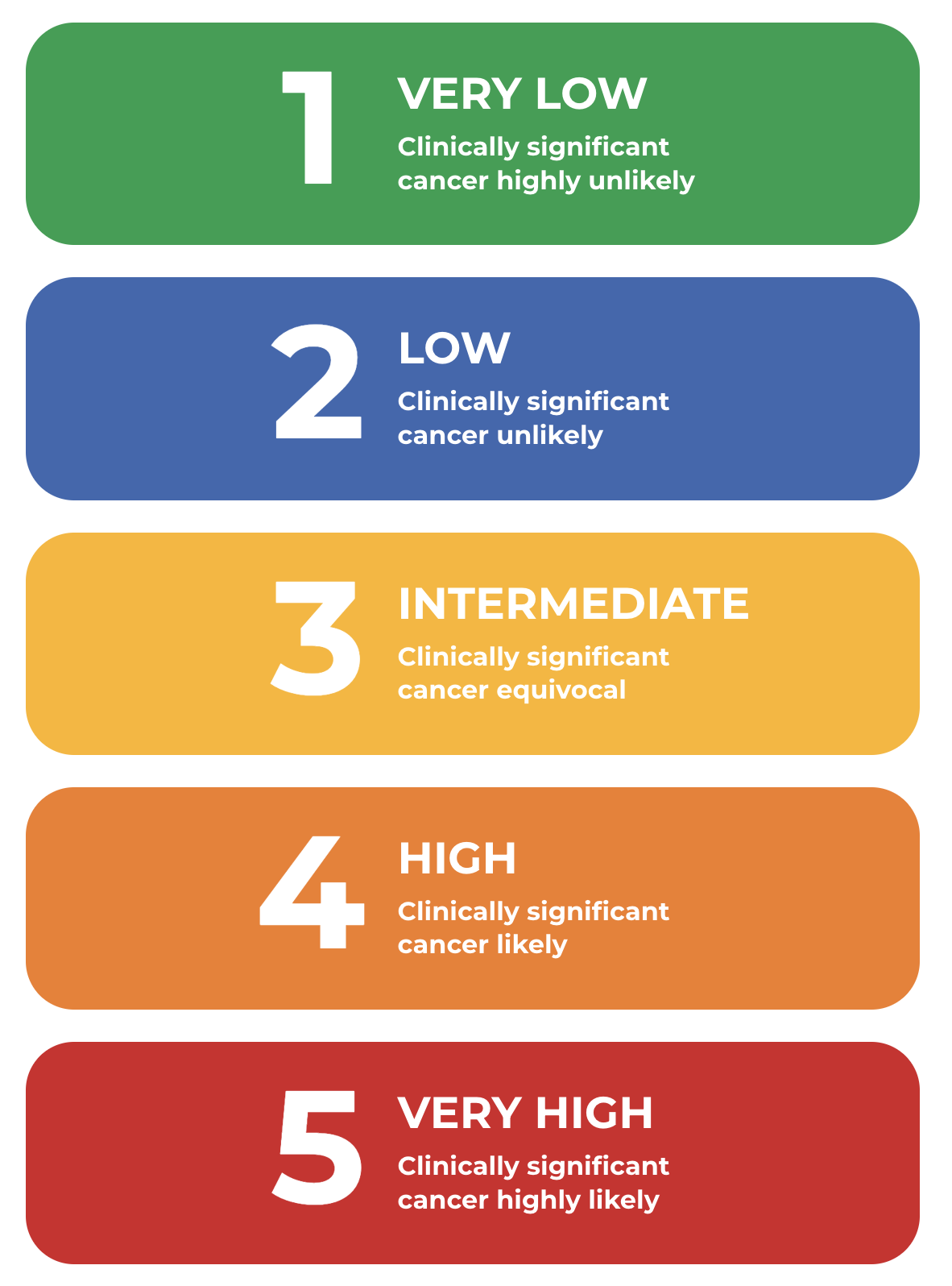
Determining a PI-RADS score is challenging, assessing lesions requires input from multiple metrics and sources of information and can be dependent on observer skill and experience. Acquiring the most accurate PI-RADS score involves determining the lesion location, whether it’s in the peripheral or transitional zone.¹
PI‑RADS Scores vs. Gleason Scores: What’s the Difference?
PI-RADS scores differ from Gleason scores in a number of ways. While PI-RADS scores are concluded by radiologists after reviewing MRI scans and enables them to assess the risk of clinically significant PCa, Gleason scores are determined by pathologists after examining biopsy specimens, and indicate the overall degree of aggressiveness of any cancer that is found. This helps inform patient management and prognosis.
How Artificial Intelligence (AI) Software is Helping Radiologists Locate Lesions
AI software is now available to help the radiology community with PI-RADS scoring as well as maintaining the current standards for PI-RADS classifications, functioning as a decision tree for radiologists to follow.
Other AI software is also providing radiologists with an alert when a particular issue might have been overlooked or had potentially been misinterpreted—when compared with PI-RADS guidelines.
Moreover, some AI software is helping radiologists determine if a suspicious area resembles a tumor and can
help evaluate the vascularity of the area.
AI Software Is Helping With PI‑RADS Lesion Classification
Radiologists are also using AI software to assist their assessment of PI-RADS classification through a program that helps segment the prostate into regions and supports the radiologist in accurately locating lesions.
AI algorithms are being developed to provide a PI-RADS score directly, to improve the diagnostic performance of
PI-RADS determination. For example, the algorithm helps the radiologist determine if a PI-RADS 3 lesion is significant prostate cancer or a nontumor case.
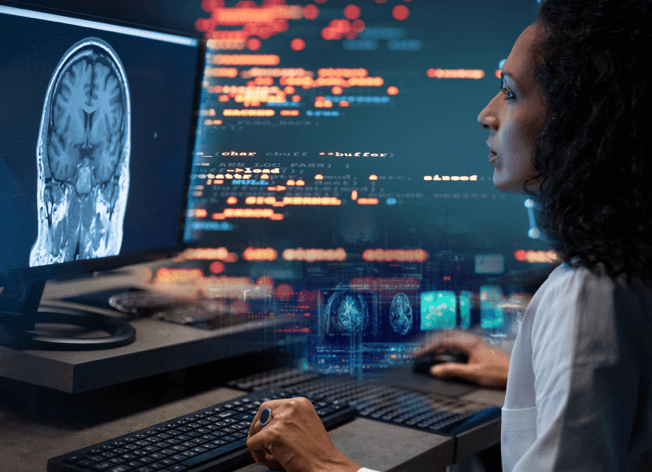
The individual who appears is for illustrative purposes. The person depicted is a model and not a real healthcare professional. All images shown are representative images from referenced studies. Individual results may vary.
AI Software is Helping Educate Radiologists on PI‑RADS
AI software has great potential to help train new radiologists on how to determine a PI-RADS score, to help boost reader agreement, and to improve the accuracy of diagnoses and treatment decisions. There is also the opportunity for AI to assist in the identification of prognostic outcomes.
Expanding the use of AI in the determination and use of PI-RADS scores specifically holds the promise of not only
simplifying the process, but minimizing the risks of human fatigue and improving the speed at which urologists and radiologists can share information. Ultimately, it’s all about bringing patients optimal care.
Recently in PCa, PI‑RADS & AI
- Deep Learning: A recent study showed that AI helped radiologists in detecting clinically significant prostate cancer in men with different demographics, showing robust results within and across datasets.2,3
- XAI: An explainable AI (XAI) model reliably detected and classified clinically significant prostate cancer and improved both confidence and reading time of nonexperts, while providing both visual and textual explanations, using wellestablished imaging features as well as the PI-RADS lexicon.4
References:
- Six O. The basics of PI-RADS and how AI can help. April 28, 2022. Accessed July 11, 2023. https://www.quantib.com/blog/the-basics-of-pi-rads-scoring-and-ai
- Hall J. Deep Learning Network Shows Significant Potential for Prostate Cancer Detection on MRI. June 22, 2023. Accessed July 11, 2023.
https://www.diagnosticimaging.com/view/deep-learning-network-shows-significant-potential-for-prostate-cancer-detection-on-mri - Hamm CA, Baumgartner GL, Biessman F, et al. Interactive explainable deep learning model informs prostate cancer diagnosis at MRI. Radiology. 2023; 307:4. Accessed July 11, 2023. https://pubs.rsna.org/doi/suppl/10.1148/radiol.222276
- Hall J. Can explainable AI enhance diagnosis and PI-RADS classification of prostate cancer on MRI? April 12, 2023. Accessed July 11, 2023. https://www.diagnosticimaging.com/view/explainable-ai-diagnosis-pi-rads-classification-prostate-cancer-mri-